Open-WebUI+QwQ-32B搭建本地知识库
一、概述
当用户提出一个问题时,如何让大模型准确的定位到你的输入背后真的正的问题,并输出正确的回复,是大模型应用的关键。 而要达到此目的,主要有三种方式:提示词、知识库和微调。
- 提示词:提示词的质量会直接影响到大模型对你的问题的定位,包括问题所属领域、背景、现象、目标等,并最终影响其输出的响应。
- 知识库:将每个不同领域的知识分别构建一个本地知识库,然后在提问时指定问题所在的目标知识库,可以让大模型的输出更加稳定,并可输出大模型底层它原本所不掌握的知识(知识库中的内容)。
- 微调: 在现有的大模型基础上,将用户自己的数据进行训练,从而让模型具备训练集里、您所特有的一些知识、内容或者风格。注:部分推理模型不支持微调。
大模型的搭建,open-webui及RAG的启用等步骤暂先跳过,本文主要介绍并演示了本地知识库的一些关键点。
二、背景
前阵子,应产品部门的要求,对Deepseek R1 671B及QwQ-32B等大模型做了一番技术上的预研。由于前期的测试中发现,在硬件受限(单卡或双卡4090)环境下,QwQ-32B-AWQ模型的表现在并发、速度等多方向优于Deepseek满血版,并且二者在会议纪要等功能的对比测试各有优劣,因此知识库的预研和测试也优先选择了QwQ-32B-AWQ模型。 而前端平台则采用了开源的open-webui,同时RAG采用了open-webui自带的“sentence-transformers/all-MiniLM-L6-v2”向量模型。
平台 | 模型 | 备注 |
---|---|---|
前端平台 | Open-webui搭建的框架 | github中开源项目,支持rag、对接ollama等功能 |
后端大模型 | QwQ-32B-AWQ | 自行部署的大模型,使用AutoDL上租借的服务器 |
向量模型 | sentence-transformers/all-MiniLM-L6-v2 | open-webui自带的向量库 |
三、影响本地知识库及响应质量的关键点
在明确了大模型(QwQ-32B-AWQ)和向量库(sentence-transformers/all-MiniLM-L6-v2)后,整个RAG应用的开发关键在于本地知识的整理和提示词的设计,在open-webui上可以看到相关的一些设定。
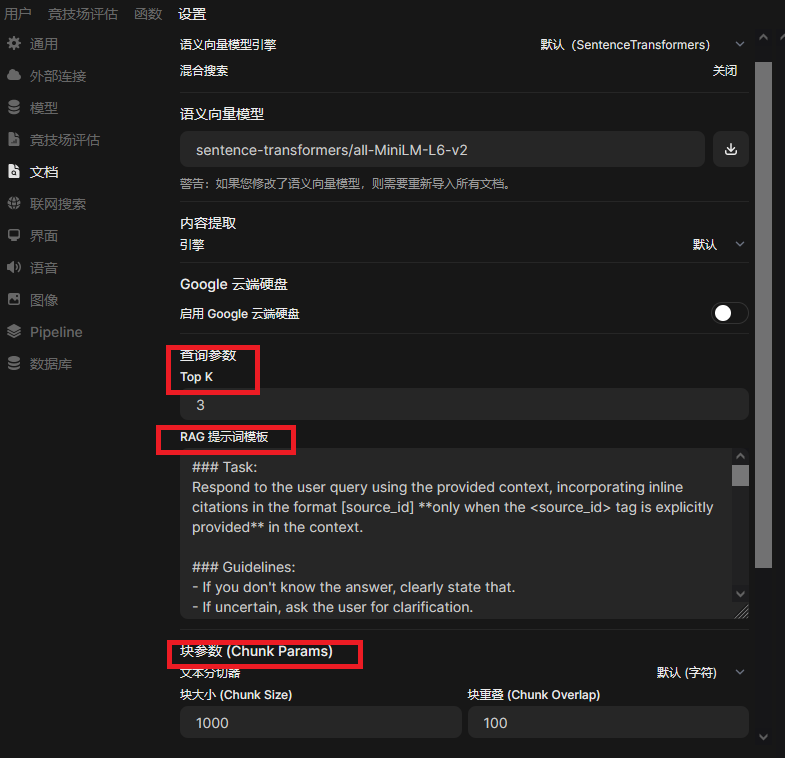
1. top k
Top-k 采样是自回归生成(autoregressive generation)“贪心策略”的优化。原理是从概率排名最高的K个单词里随机采样。很多情况下这个随机性有助于提高生成质量。默认为前3。
2. 提示词
open-webui给出了一个样例的提示词。
### Task:
Respond to the user query using the provided context, incorporating inline citations in the format [source_id] **only when the <source_id> tag is explicitly provided** in the context.
### Guidelines:
- If you don't know the answer, clearly state that.
- If uncertain, ask the user for clarification.
- Respond in the same language as the user's query.
- If the context is unreadable or of poor quality, inform the user and provide the best possible answer.
- If the answer isn't present in the context but you possess the knowledge, explain this to the user and provide the answer using your own understanding.
- **Only include inline citations using [source_id] when a <source_id> tag is explicitly provided in the context.**
- Do not cite if the <source_id> tag is not provided in the context.
- Do not use XML tags in your response.
- Ensure citations are concise and directly related to the information provided.
### Example of Citation:
If the user asks about a specific topic and the information is found in "whitepaper.pdf" with a provided <source_id>, the response should include the citation like so:
* "According to the study, the proposed method increases efficiency by 20% [whitepaper.pdf]."
If no <source_id> is present, the response should omit the citation.
### Output:
Provide a clear and direct response to the user's query, including inline citations in the format [source_id] only when the <source_id> tag is present in the context.
<context>
{{CONTEXT}}
</context>
<user_query>
{{QUERY}}
</user_query>
这个提示词本身已经经过了许多人的检验,理论上讲应该适用于大部分的场景,但暂未在公司的使用场景下做严格测试和验证。未来我们可以在使用中观察一下,并根据实际的请求与响应来做一下各种必要的调试或调整。
3. 块参数(Chunk Params)
包括:
- 块大小(Chunk Size):影响检索的效率,越小,检索速度越慢。
- 块重叠(Chunk Overlap):影响文档知识丢失的概率。
向量化参数块大小和块重叠的设置,这直接影响了rag检索的效果。推荐:块大小1000,块重叠为块大小的5%-10%,若发现知识丢失,可适当增加块重叠的值。
四、创建和使用知识库
创建知识库
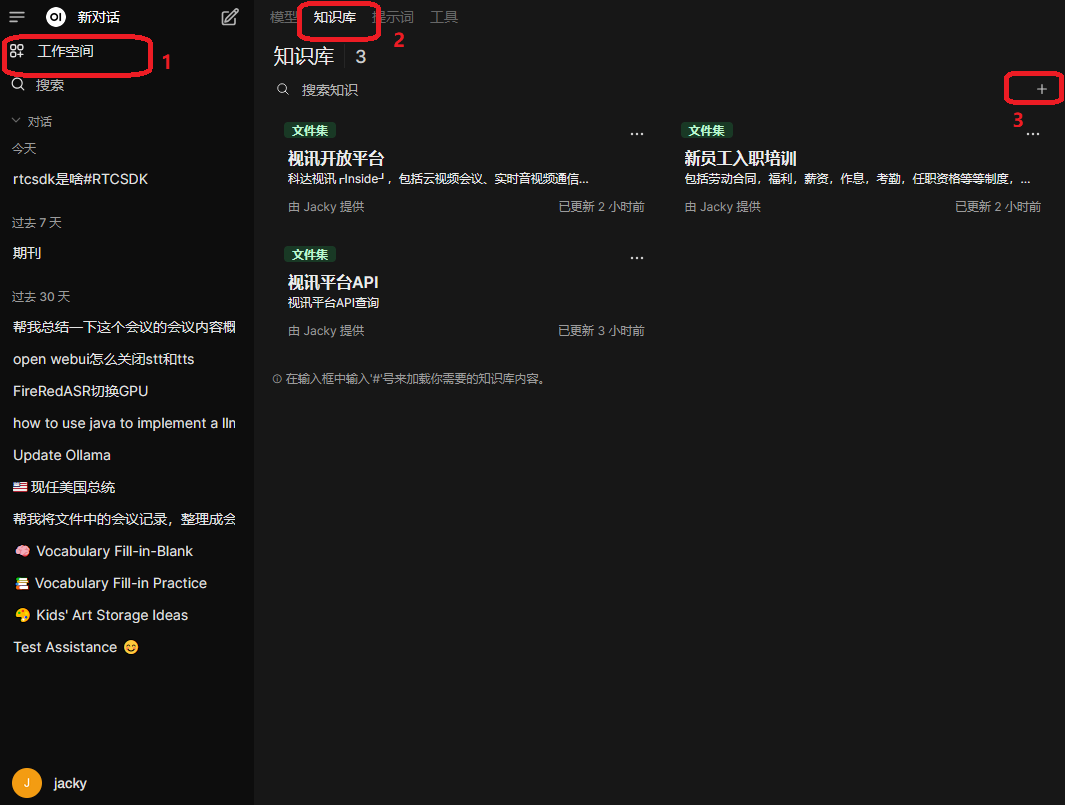
知识库的创建步骤,如上图所示:
- 选择工作空间(Workspace)
- 选择知识库(Knowdge)
- 点右侧的”+”号新增知识库(可以是个人私有的,也可以是公开的)
知识库使用介绍
知识库创建好了之后,到了主界面,在输入框里输入一下 #,你就可以看到所有你具体访问权限的知识库列表,选定你要问的知识库后,再在输入框里输入你的问题,即可针对知识库来进行问答。
五、演示环境
目前我在演示环境建了三个知识库,视讯开放平台,新员工入职培训,视讯平台API。 大家可以实际体验一下效果和准确率。
地址:http://172.16.129.127:3000 测试账号: admin1@localhost 123456 admin2@localhost 123456 user1@localhost 123456 user2@localhost 123456